KIbaroP
AI-based robust production planning
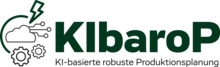
The aim of the 'KIbaroP' research project is to develop and validate an AI-based and robust approach to production planning with special consideration of SME-specific data.
Initial Situation
In manufacturing companies, the improvement of disruption avoidance plays a central role against the background of increasing susceptibility to disruptions in production. In industrial practice, disruptions are currently not taken into account in production planning, or only through estimated surcharges (e.g. 10 to 20 percent of the throughput time) on the planned throughput time. These surcharges are used in an attempt to increase adherence to deadlines despite any disruptions that may occur. However, there is a risk that excessively long lead times will lead to reduced customer satisfaction, higher storage costs and reduced productivity.
Expected Result
A robust approach to production planning based on artificial intelligence (AI) is being developed and validated, with special consideration given to the specific challenges of small and medium-sized enterprises, such as limited data availability:
- Inclusion of disruptions in the areas of personnel, materials, equipment, warehouse, process and IT in production planning
- Use of existing production data and aggregation with operational system data
- Inclusion of historical and current feedback data to assess the risk of disruption
- Processing the information obtained using AI approaches to identify critical jobs or workplaces
Solution Approach
The solution is based on the industry-oriented process CRISP-DM (Cross Industry Standard Process for Data Mining) in order to develop a practical solution as a software demonstration application for small and medium-sized companies. This application takes into account production data, feedback data and other data from the operational system landscape and the socio-technical environment. The data available for training the AI algorithms forms the basis for the application of AI approaches.
- Business understanding: structured literature research and conducting expert interviews on the topics of disruption management, production planning and control
- Data Understanding: explorative data analysis of provided company data, development of a generic simulation model to generate synthetic data
- Data preparation: data cleansing, creation of a standardized database
- Modeling: Building an AI model to predict disruptions
- Evaluation: Review of the results with the consortium partners
- Deployment: Implementation of AI model in demonstrator, integration of AI model in production planning of the consortium partners
Benefits for the Target Group
The development of AI-based and robust production planning offers companies both direct and indirect benefits. The direct benefit lies in the creation of an understanding of disruptions, which enables preventive measures to be taken in production planning. In addition, the existing knowledge of production data is further deepened and its preparation for AI applications is described. It also shows how insights can be generated from disruption data and used preventively.
The indirect benefit is the long-term increase in competitiveness through higher delivery reliability and customer satisfaction. The sustainable increase in productivity through reduced disruption enables companies to increase their profitability.
Research partners
Project partners
- electronic service willms GmbH & Co. KG, Stolberg-Breinig
- GKD – Gebr. Kufferath AG, Düren
- INFORM Institut für Operations Research und Management GmbH, Aachen
- Jochen Günther Training & Consulting, München
- MunichRe, München
- ONIQ GmbH, Köln
- Phiesel GmbH, Bad Münstereifel
- PSI Automotive & Industry GmbH, Berlin
- Rausch Druck GmbH, Augsburg
- REO AG, Solingen
- Robert Josef Wolf GmbH & Co. KG, Wilnsdorf
- Schoeller Werk GmbH & Co. KG, Hellenthal
Topic Area
- Production Management
Research Focus
- Produktionsregelung
FIR Topics
JRF Guiding Topic
- Society & Digitization
- Industry & Environment
Contactperson
Projectinfos
Duration
Funding no.
01IF23054 NFunding information
This pre-competitive project was funded by the Federal Ministry for Economic Affairs and Climate Action with IGF funds.